科技期刊
在线办公
一种融合KPCA和BP神经网络的用水总量预测方法-赵和松 ,王圆圆 ,赵 齐
摘要:为提高用水总量预测的准确性,针对现有方法中存在的非线性多维用水因子选取不合理的问题,提出一种融合KPCA(核主成分分析)和BP神经网络的用水总量预测方法。
一种融合KPCA和BP神经网络的用水总量预测方法-赵和松 ,王圆圆 ,赵 齐
摘要:为提高用水总量预测的准确性,针对现有方法中存在的非线性多维用水因子选取不合理的问题,提出一种融合KPCA(核主成分分析)和BP神经网络的用水总量预测方法。
分类:2021年第05期(总第164期)
发布: 2021-11-10 21:15:25
赵和松1 ,王圆圆2 ,赵 齐3
(1. 水利部信息中心,北京 100053;2. 北京金水信息技术发展有限公司,北京 100053;3. 河海大学计算机与信息学院,江苏 南京 211100)
摘 要:为提高用水总量预测的准确性,针对现有方法中存在的非线性多维用水因子选取不合理的问题,提出一种融合KPCA(核主成分分析)和BP神经网络的用水总量预测方法。使用皮尔逊相关系数对用水因子进行相关性分析,选择与用水总量最相关的多个因子作为数据输入,利用KPCA对预测因子进行降维处理,解决数据间的非线性特征,再采用BP神经网络建立用水总量预测模型,网络的权值和阈值采用思维进化学习算法进行调优。采用国家统计局2007—2016年度开放统计用水数据进行实验,结果表明:融合KPCA和BP的用水总量预测方法相对预测误差小于5%,预测用水精度有明显提升,可以较好预测未来用水总量,为高维非线性数据的预测提供新的优化思路。
关键词:用水总量预测;KPCA;BP神经网络;思维优化算法;融合方法
Water consumption prediction approach using KPCA and BP neural network
ZHAO Hesong1, WANG Yuanyuan2, ZHAO Qi3
(1. Information Center, Ministry of Water Resources, Beijing 100053, China;
2. Beijing Jinshui Information Technology Development Co., Ltd., Beijing 100053, China;
3. College of computer and information, Hohai University, Nanjing 211100, China)
Abstract: In order to improve the accuracy of total water consumption prediction, aiming at the unreasonable selection of nonlinear multi-dimensional water consumption factors in existing methods, a total water consumption prediction method based on KPCA (kernel principal component analysis) and BP neural network was proposed. Pearson correlation coefficient is used to analyze the correlation of water consumption factors. The most relevant factors of total water consumption are selected as data input. KPCA is used to reduce the dimension of prediction factors for solving the nonlinear characteristics of data. BP neural network is used to establish the prediction model of total water consumption. The weights and thresholds of the network are optimized by mind evolutionary learning algorithm. Using the water consumption data from the State Statistics Bureau between 2007 and 2016 for experiment, of which the results show that relative prediction error of the method based on KPCA and BP is less than 5%, and the prediction accuracy is significantly improved, which can better predict the future total water consumption, and provide a new optimization idea for the prediction of high-dimensional nonlinear data.
Key words:Total water consumption prediction; KPCA; BP neural network; mind evolutionary algorithm; fusion approach
-
一种融合KPCA和BP神经网络的用水总量预测方法.pdf下载下载量:0
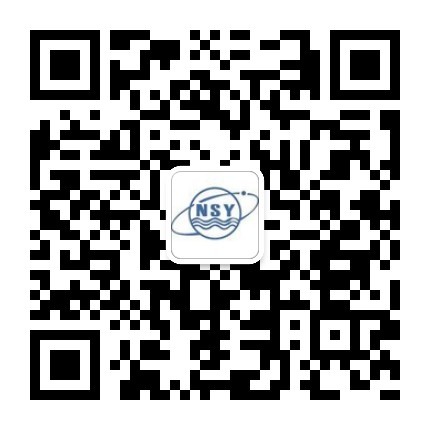
水利部南京水利水文自动化研究所
电话:(025)52898300
地址:南京市雨花台区铁心桥街95号
邮箱:nsy@nsy.com.cn
版权所有:水利部南京水利水文自动化研究所 苏ICP备05086125号 中企动力 南京